Here are some of our unique training courses developed at the AI4Health CDT:
✍️📚 Bespoke training to develop critical thinking, presentation, and writing skills:
“Research Tutorials in AI & ML for Healthcare”
As a PhD student, it’s important to develop a range of skills such as critically evaluating research papers, delivering clear talks and presentations, and improving academic writing proficiency. At the AI4Health CDT, we pioneered a unique module called the Research Tutorials designed to help students build up skills which will be critical during their research journeys.
The Research Tutorials follow a reading group style where first-year PhD students convene every week to review and discuss an interesting research paper on AI and/or healthcare. One of the students takes the lead every week with presentation and writes a summary report that outlines the main points of the paper, as well as the discussion that took place.
Crucially, we strive to improve the module continuously, and have recently introduced a peer-feedback component to encourage a culture of collaborative research. As part of this component, each student provides the presenter with immediate constructive feedback regarding what they liked about the presentation and what they would do differently.
Through this approach, we aim to foster a supportive environment where students can openly discuss new ideas and receive constructive feedback.
The students also receive formal personalised feedback on the summary report from the module leader, helping students identify areas for improvement in their academic writing early on in their research journey.
Here’s a selection of summary reports written by our students on a number of interesting papers:
— Daolong Chen (Cohort 5) summarising the NeurIPS paper “Attention Is All You Need”
— Ollie Pitts (Cohort 5) summarising the Cell paper “A Deep-Learning Approach to Antibiotic Discovery”
🤖 🏥 Regulatory training on software as a medical device with focus on AI:
“Regulation and Innovation”
Developing clever algorithms is only one part of the wider picture of innovation in AI for healthcare. It is important to appreciate medical technology regulations and laws, patient safety and needs, R&D project success and failures, as well as wider organisational changes. These are relevant regardless of whether our PhD students will continue to innovate in academia, as part of the NHS, in start-ups, or within large organisations.
At the AI4Health CDT, we run a yearly regulatory course where experts from the industry and academia give our students an overview of what it takes to regulate an innovation in healthcare.
Upon completion of this programme, students are expected to:
1. Demonstrate an understanding of how regulatory processes work for medical devices, including AI Software as a Medical Device (SaMD).
2. Develop an awareness of the clinical regulatory landscape in Britain and the EU, in the context of UKCA and CE marking.
3. Appreciate the importance of building AI with highest levels of safety, and demonstrate ways in which this can be achieved in healthcare.
4. Identify ways to pioneer in the health AI space and become aware of obstacles innovators face.
5. Present and defend a solid proposal to technology experts in the form of a product pitch.
🖥️ 🩺 Self-paced training for clinical researchers:
“Introduction to AI for Clinical Researchers”
The AI4Health CDT has developed a new online eLearning course on AI: “Introduction To Artificial Intelligence For Clinical Researchers”.
The course was developed in partnership with the NIHR Clinical Research Network (CRN) and Imperial’s Institute of Global Health Innovation (IGHI), and aims to build awareness and knowledge in AI for professionals developing and carrying out research.
The material is self-paced and was designed for clinical researchers that could benefit from AI methods, including research managers, research nurses, clinicians, and researchers.
The course is available on the NIHR Learn platform to NHS staff and UK universities, and over 200 users have already enrolled in the course.
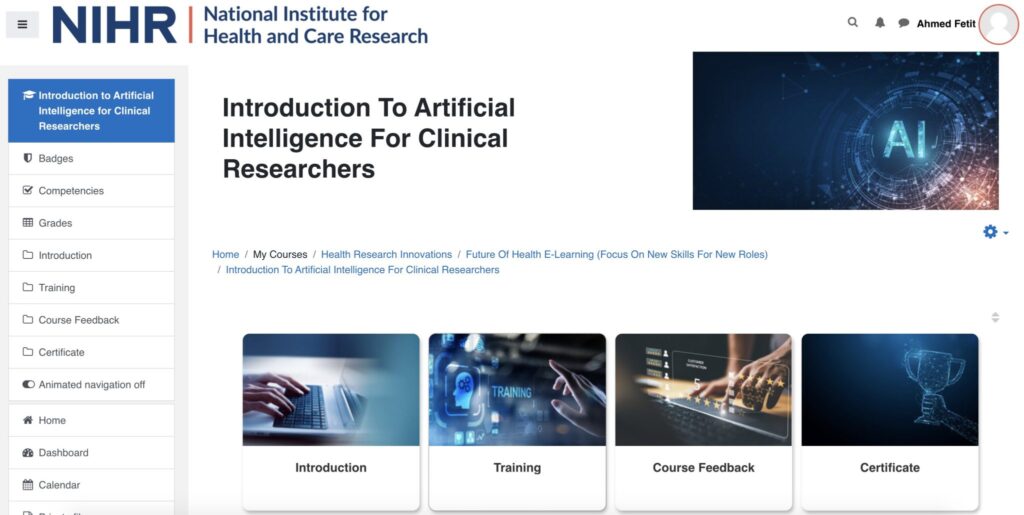